CLINICAL STUDIES
Objective Symptom Measurement for
Patients with Parkinson’s
ConnectedLife and National Neuroscience Institute Singapore have collaborated in an observational, non-interventional study to determine the validity of an IMU (Inertial Measurement Unit) sensor-based approach. We objectively assess motor fluctuations and motor/non-motor symptoms in Patients with Parkinson’s Disease (PwP).
Parkinson’s Disease (PD) is characterized by a loss of spontaneous movement that is dramatically alleviated by dopamine restitution. The dopamine precursor levodopa – after 50 years of use – is still considered to be the dopaminergic compound with strongest therapeutic potency, as well as superior safety. However, long-term treatment frequently leads to symptom fluctuations, which are inadequately addressed by impersonalized and rigid timing schedules for drug administration.
Managing motor fluctuations successfully is one of the greatest challenges of modern PD therapy. Recently, the description of motor symptoms in patients with PD has been disrupted by the introduction of body-worn sensors, which describe the PD motor syndrome with objective parameters for the first time. This surge of new low-cost hardware devices can reliably detect bradykinesia, tremor, postural disturbance and freezing of gait promising continuous home-based monitoring of PD symptoms 1-5. Modern technology has enabled patients to carry sensors over longer periods of time and in remote environments, allowing a multitude of motor parameters to be described in the absence of placebo effects and rater bias.
PD’s transformation and intensive levodopa therapy
The advent of objective and seamless measurement of parkinsonism will help close the feedback loop between clinical assessment and pharmacological treatment. Furthermore, with the help of data science, biomedical algorithms could even be predictive by recognizing patterns that evade the clinical assessment of doctors. We believe that pharmacotherapy with dopaminergic for patients with Parkinson’s disease is just short of undergoing a similar transformation, like insulin treatment did in previous decades.
In the study with the National Neuroscience Institute Singapore, we developed and refined an objective measure for motor fluctuations in PD patients and target objective measurement of specific PD symptoms. The patients are rated by their doctors using clinical rating scales (UPDRS, MOCA etc.). We use a single wrist-worn device to collect supervised and free-living motion data. Furthermore, we test user experience and gather feedback from patients to develop methods and applications for automated motor state monitoring.
With a sample of 100 patients, we are in the midst of acquiring 3000 minutes of supervised expertly labeled motion data (with additional retrospective video assessment) and more than 100x of unsupervised free-living motion data. The raw data is then processed and used to develop a predictive classification model of PD motor state, and detect specific PD motor symptoms. We have performed previous studies in the field of motion sensing in Parkinson’s Disease which has been achieved in a test-based as well as free-living setup, and serves as profound scientific basis for this research study.
For more information on our clinical studies on Parkinson’s Disease, please see below:
Leveraging technology for timely and effective intervention
What we are aiming to achieve is >90% accuracy for the scoring from the device compared to an expert rater, using pre-trained models, algorithms to help administer medication levels, pumps and determine suitability for Deep Brain Stimulation (DBS) surgery. Labelling by human experts (UPDRS scoring) only give you 78% accuracy as a result of small sample and can be extremely time consuming. PwP patients’ self-labelling will improve model accuracy to 85%. Data captured by sensors (large scale and unlabelled data), building algorithms and machine learning from the experts’ observations and PwP patients’ own labelling will improve model accuracy further and above the 90% acceptable requirement. Wearing the wrist-watch for 10-12 hours a day (daylight and awake hours) allows for live-monitoring of the PwP & real-time collection and analysis of data and objective scoring. This is helping us to identify or predict an episode occurring based on the trained models. An example of this would be Freezing of Gait or Rigidity which are major concerns. With an alert sent to the patient (alarm, vibration or sound to snap the PwP out of the freezing situation) and notification to the caregiver, we aim to prevent falls and further injury to the PwP. An app to support day-to-day living for the PwP with reminders for medication and exercise, rest indication and inputs for self-labelling, can provide further insights to the PwP’s day.
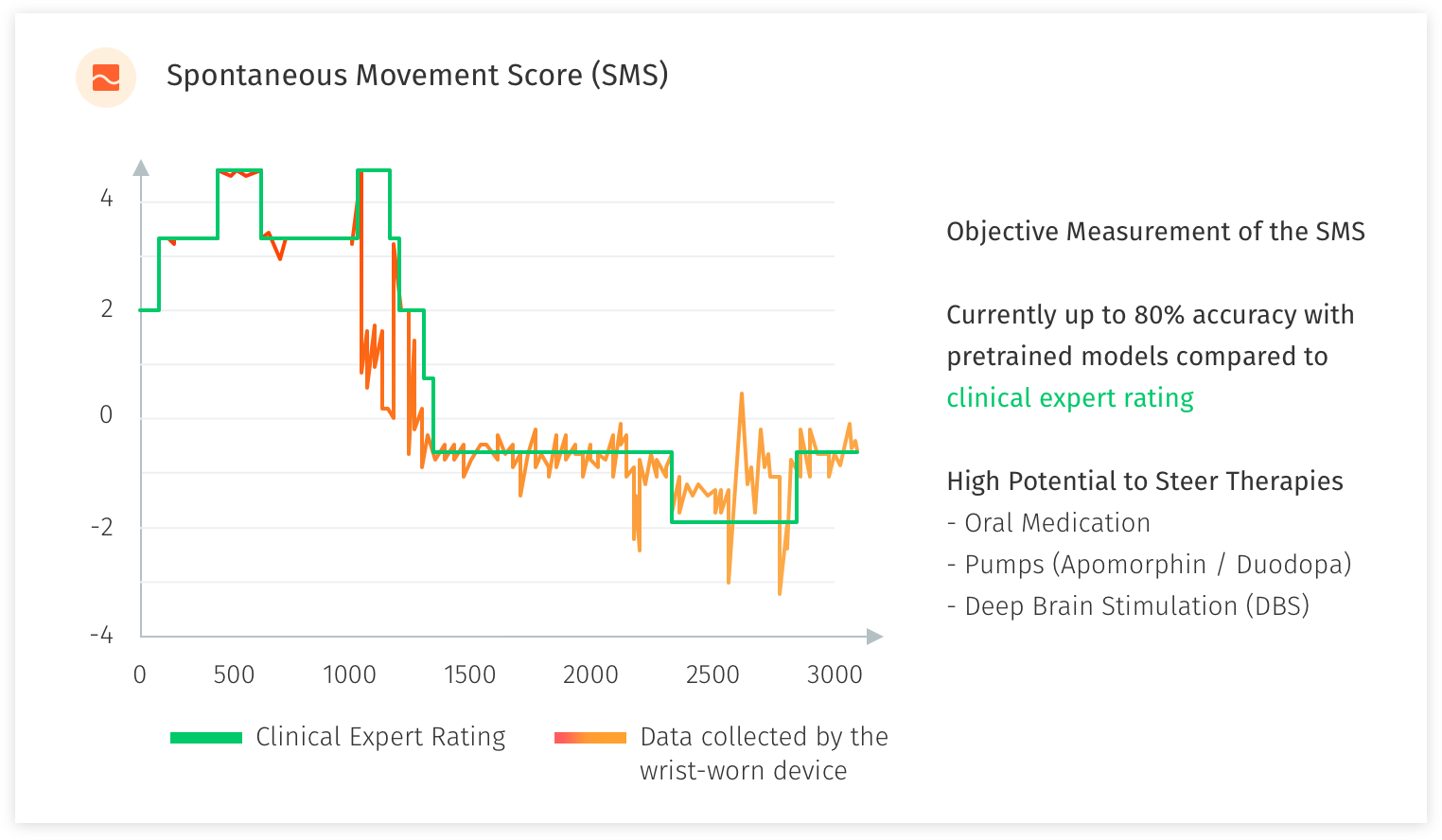
References
- Smith SL, Lones MA, Bedder M, Alty JE, Cosgrove J, Maguire RJ, et al. Computational approaches for understanding the diagnosis and treatment of Parkinson’s disease. IET Syst Biol. 2015 Dec;9(6):226–33.
- Kassavetis P, Saifee TA, Roussos G, Drougkas L, Kojovic M, Rothwell JC, et al. Developing a Tool for Remote Digital Assessment of Parkinson’s Disease. Mov Disord Clin Pract. 2016 Jan;3(1):59–64.
- Lockhart TE, Soangra R, Chung C, Frames C, Fino P, Zhang J. Development of automated gait assessment algorithm using three inertial sensors and its reliability. Biomed Sci Instrum. 2014;50:297– 306.
- Horak FB, Mancini M. Objective biomarkers of balance and gait for Parkinson’s disease using body-worn sensors. Mov Disord Off J Mov Disord Soc. 2013 Sep 15;28(11):1544–51.
- Yang K, Xiong W-X, Liu F-T, Sun Y-M, Luo S, Ding Z-T, et al. Objective and quantitative assessment of motor function in Parkinson’s disease-from the perspective of practical applications. Ann Transl Med. 2016 Mar;4(5):90.